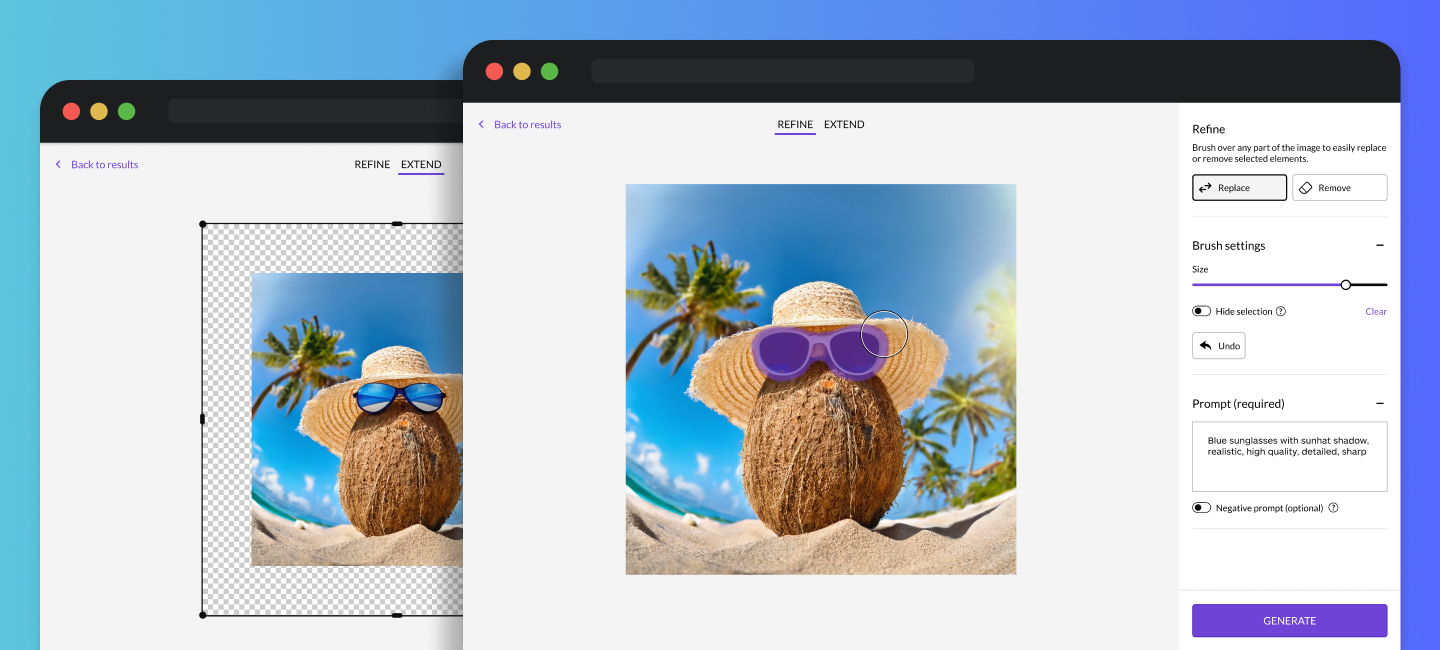
About the project
Getty Images and iStock are trusted platforms for creative professionals looking for high-quality visual content. As the company expanded into generative AI, we introduced an image generator that allowed users to create visuals from text prompts, however, the initial version of the product offered little flexibility after image generation.
As Lead Designer on this project, I was responsible for partnering closely with our cross-functional team to define and deliver new editing capabilities. I helped drive the design direction, ensured we stayed aligned on priorities, and provided mentorship and support to other designers. I also played a key role in maintaining momentum and ensuring we hit our deadlines and goals.
Together, we launched two new features:
• Refine (inpainting): Edit or remove specific parts of an image
• Extend (outpainting): Expand an image in any direction to “zoom out” or reframe
These tools helped users go beyond prompt iteration and fine-tune their images, all without needing advanced editing skills.
My role: Lead Designer
Team: Cross-functional group including other product designers, content design, research, PM, PO, developers
Platforms: GettyImages.com, iStock.com
Timeline: ~6 months (from discovery to first release)
The opportunity
AI image generation is powerful but often unpredictable. Users struggled to get to their ideal image, even after multiple generations. Common frustrations included:
• Common quality issues in generated images (ex. distorted hands)
• Difficulty achieving the right framing
• Unwanted elements or missing elements
• A lack of creative control
We heard this consistently in user research and saw it reflected in product analytics. Feedback often described the experience as “like pulling a slot machine handle.”
This was a clear opportunity to help users shape their images more intentionally, especially those without the time, skill, or software to edit visual content.
Discovery and prioritization
While generative AI technology was advancing rapidly, we made an intentional decision not to throw every available capability into the product. Our team had access to a wide array of potential features, from sketch-to-image to outpainting, but our goal was to focus on the tools that would be most impactful for our audience.
To identify the right opportunities, we:
• Conducted surveys and qualitative interviews
• Partnered with our sales and support teams to gather feedback
• Monitored the competitive landscape to identify emerging expectations
• Tested AI editing behaviors directly using internal tools and NVIDIA’s model playground
I co-led a cross-functional workshop with PMs, researchers, data scientists, and engineers to collect potential use cases, then facilitated a prioritization exercise based on:
• Customer value
• Technical feasibility
• Alignment with NVIDIA’s roadmap (as they powered our generative model)
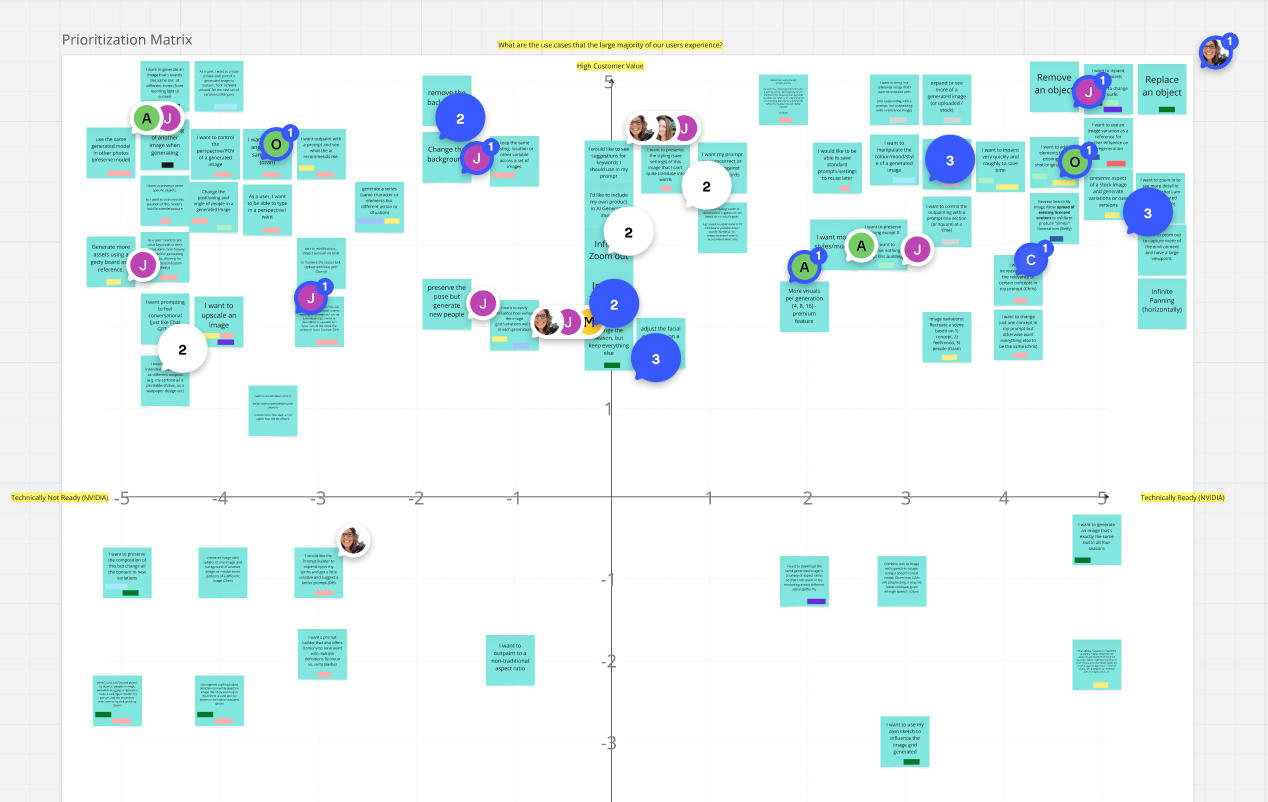
Ultimately, we landed on Refine(inpainting) and Extend(outpainting) as our first features; two clear, highly-requested capabilities that directly addressed user pain points and mapped cleanly to both business and user goals.
Designing for everyday users
One of the biggest challenges was ensuring that what we designed was not only technically possible but also intuitive and effective for users who weren't experts in generative AI.
I worked closely with internal AI experts and our partners at NVIDIA to understand the capabilities (and limitations) of the model. For example, with Extend, we weren’t initially sure if users could expand an image in multiple directions simultaneously (e.g. top and left). I tested the model to explore edge cases and advised the team on where user expectations might exceed what was realistically achievable.
Alongside technical feasibility, we focused heavily on usability, especially for less experienced AI users. We intentionally avoided technical jargon like “inpainting” and “outpainting,” choosing instead names and descriptions that communicated what the tools did and the value they provided.
Workflow and wireframing
Rather than design these features in isolation, we took a workflow-first approach. The goal was to create a seamless editing experience, not a series of disconnected tools.
In the early wireframing phase, we explored how a user might:
1. Generate an image
2. Notice something off or missing
3. Launch Refine or Extend to adjust
4. Preview results and download
We made sure users didn’t have to “switch modes” or feel like they were jumping into a separate app. Every design decision was made to reinforce the flow, not fragment it.
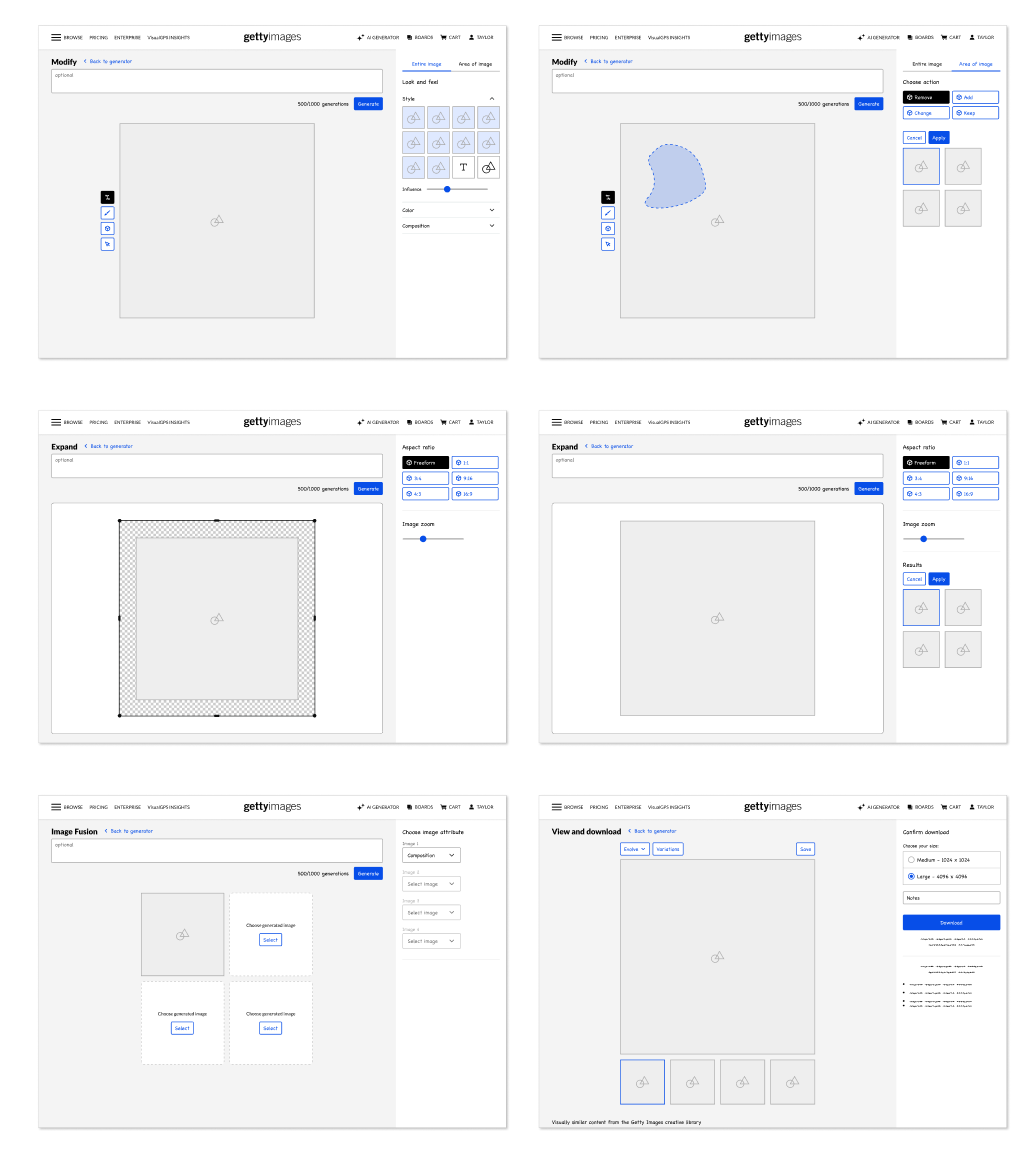
High-fidelity prototypes and user testing
Once aligned on direction, I led the transition into high-fidelity design, along with my fellow product designer and content designer. We partnered with our researcher to test a prototype that covered:
• Tool discovery and navigation
• UI clarity and labeling
• Value perception of each feature
This study also included early explorations of the Reference Image feature—our next focus—to ensure a cohesive editing experience across the board.
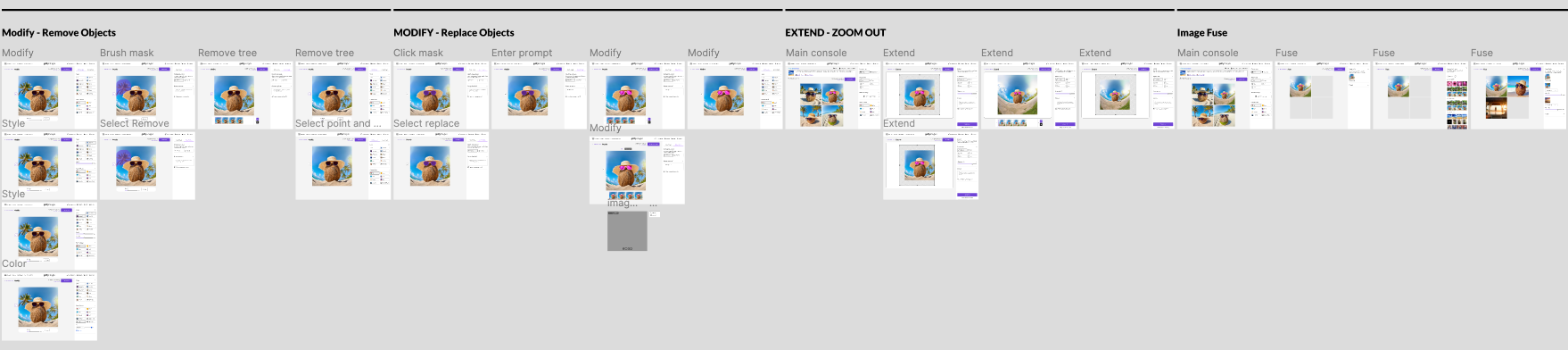
Onboarding and education
Since these were unfamiliar concepts for many users, I advocated for onboarding support early on. Informed by insights from our competitive audit and user testing, I designed:
• Discovery callouts to encourage users to engage with the feature
• Short animations that quickly demonstrated each feature’s purpose and behavior
• Contextual tooltips to guide first-time users through the workflow
This helped users understand not just how to use the features, but the value behind them.
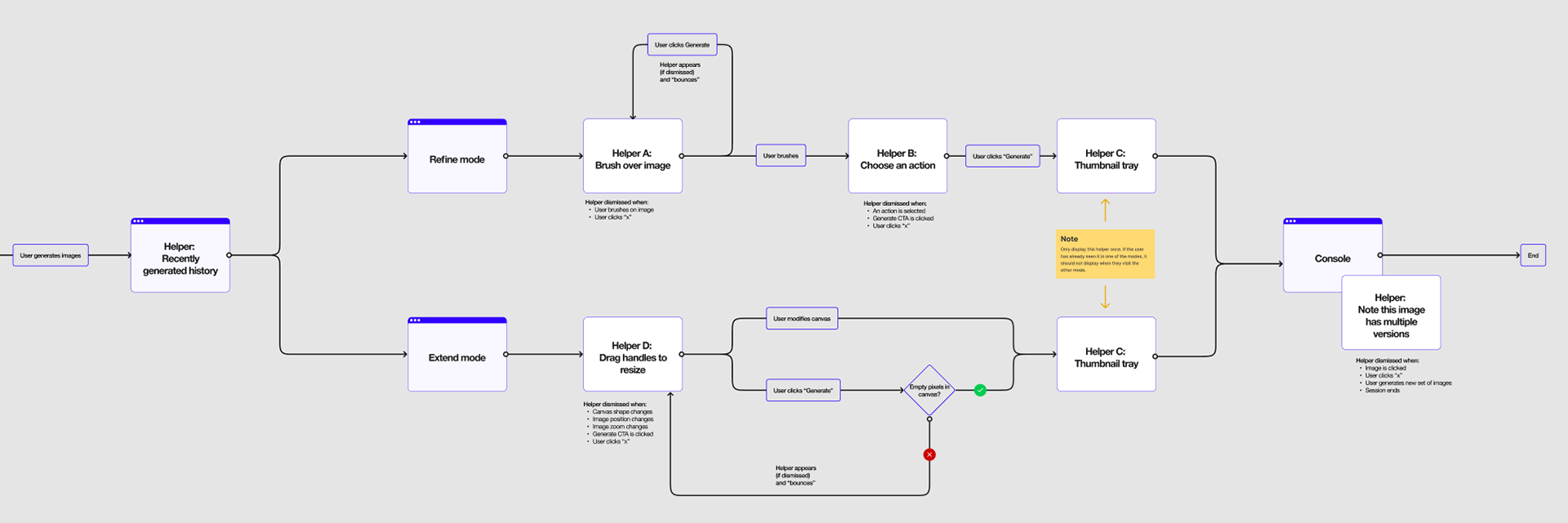
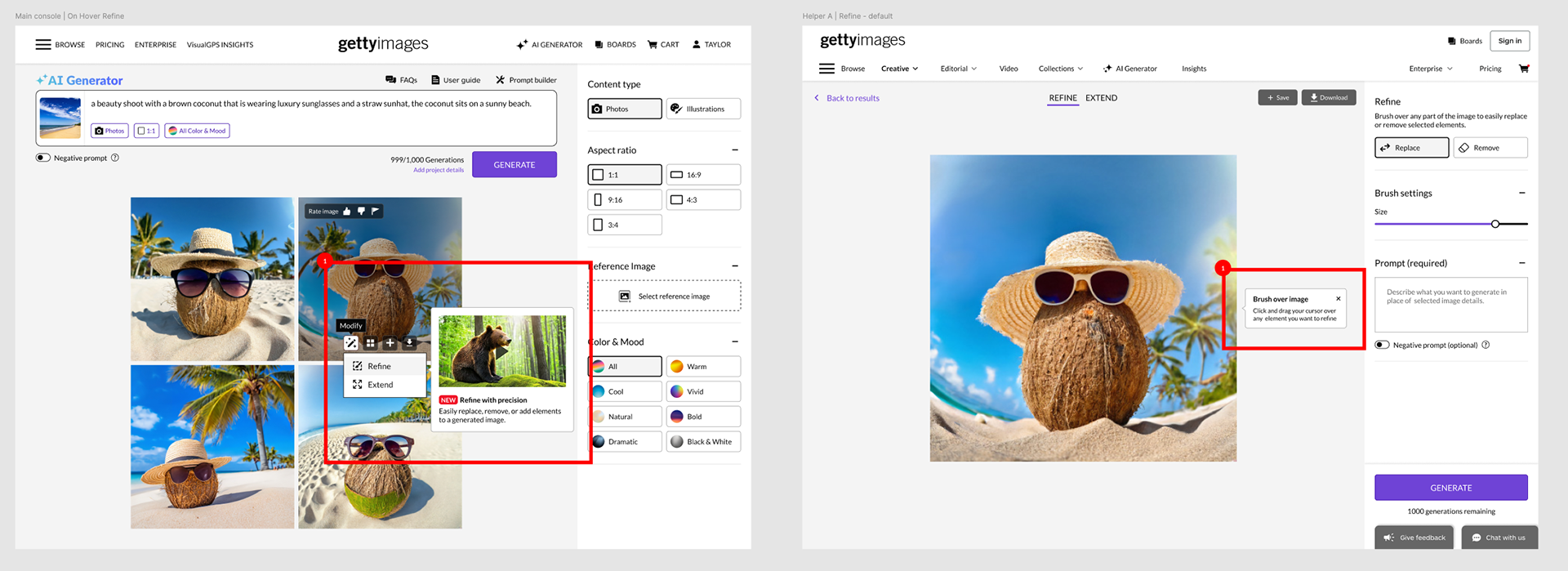
The Final Experience
We launched Refine and Extend first for generated images, allowing users to fix or customize their output without restarting. Shortly after, we expanded both tools to work on pre-shot creative images in Getty Images and iStock’s libraries, something we planned for from the beginning. Because we approached the design with this broader use case in mind, the transition was fast and smooth, leading to wider adoption and greater business impact.
The final solution gave users a seamless, intuitive way to modify their images without disrupting their flow. Refine and Extend were fully integrated into the existing generation workflow, allowing users to fix specific areas or expand their image without needing to start over or switch contexts.
Micro-animations played a key role in making the workflow feel smooth and continuous. Users could see their image move from generation into editing, then back to preview, helping reinforce control without adding complexity.
Impact
While the business goals were intentionally left open-ended given the rapidly evolving nature of the space, the features contributed to increased engagement and retention. Notable outcomes included:
• Enabled a new editing workflow for both generated and stock imagery
• Drove increased generation volume and product engagement
• Supported growth in generative AI agreements
• Contributed to customer retention and satisfaction